Recently updated on July 29, 2024
For some, a mention of machine learning summons scenes from sci-fi movies like Ex Machina, where robots conquer people with their unlimited intellect. But the reality is that today’s ML – the ability of machines to learn from experience and perform heavyweight tasks instead of humans – is already a reality full of possibilities to enrich user experiences in many areas of human life, from reading mailbox to managing personal finances.
Machine learning revolutionised the Finance and Banking industries – from public relations to investment decisions. But how exactly can Fintech companies incorporate this innovative technology in their products to drive results? We will discuss 7 top machine learning use cases in the Finance and Banking industries to shed light on the opportunities ML provides for Fintech and some challenges to overcome.
Stay tuned. This article will be read much faster by robots than by humans.
In this article, we’ll discuss:
- Quick facts about machine learning in the finance industry
- Machine learning for fraud detection
- Machine learning for Anti-Money Laundering
- Machine learning for meeting compliance regulations
- Machine learning for customer retention
- Machine learning in stock market forecasting
- Machine learning for investment strategies
- Machine learning for risk assessment
- Final thoughts
- FAQ
Quick Facts About Machine Learning in the Finance Industry
But before we dive into the real-life cases of the application of machine learning in banking and finance, let’s look at some stats. The main takeaway from the analytical reports is simple: Machine learning in financial services is maturing, bringing the potential for higher-complexity solutions that generate positive ROI across business segments.
Here, read some facts we’ve highlighted:
Fact #1: adopting AI and ML solutions in Finance is becoming mainstream. According to the Cambridge Centre for Alternative Finance and the World Economic Forum, many financial services companies report incorporating technology in risk management (56%) and revenue generation through new products and processes (52%).
Fact #2: 48% of surveyed US bank executives plan to use genAI to enhance customer-facing chatbots and virtual assistants, per an October 2023 Google Cloud survey. This increased exposure will drive further consumer adoption of interactive chatbots.
Fact #3: Implementing artificial intelligence and machine learning will be critical for financial institutions to stay competitive and thrive in 2024. Both web and mobile banking adoption among US consumers will increase, reaching 72.8% and 58.1%, respectively, due to Insider Intelligence.
What we’ve also noticed is that ML technology is being rapidly implemented in the banking sector – 75% of respondents at banks with over $100 billion in assets say they’re currently deploying AI and ML technologies due to Insider Intelligence. AI applications offer the most significant cost savings opportunity across digital banking.
That is precisely why we will start discussing machine learning use cases for the financial services industry with ML opportunities for banks.
7 Top Machine Learning Use Cases in Banking and Financial
Machine learning for fraud detection
As hackers get increasingly creative with their tactics, banks face increased pressure to stay ahead of criminals when fighting financial crime, especially fraud and money laundering. ML implementation for fraud detection helps banks identify malicious activity, quickly verify user identity, and immediately respond to cyber-attacks.
Machine learning algorithms can process large amounts of data in seconds. Moreover, the ability to learn from previous experience and improve models minimises human input. With ML algorithms, the system can quickly recognise suspicious activity and send alerts to the security operations centre or automatically decline the transaction in case of credit card fraud.
Besides rule-based fraud detection, ML allows the scanning of large amounts of data in real-time and minimises human involvement in the process. It also makes the user experience much better by simplifying the identity verification measures. Here, see what difference ML makes compared to rule-based fraud detection:
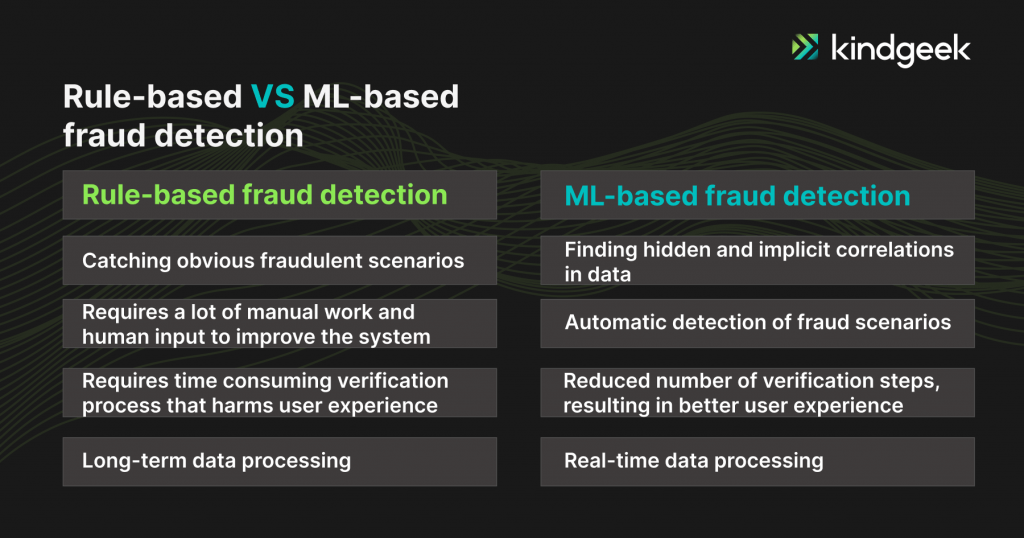
Source: AlexSoft
Here are a few notable startups that use machine learning to help businesses outsmart hackers and financial criminals:
Resistant.ai is a Prague-based machine learning-powered startup that helps to protect AI systems from targeted manipulation, adversarial machine learning attacks, and advanced fraud.
Riskified uses machine learning algorithms to provide payment insights that enable businesses to accept or reject transactions.
Feedzai is a US startup that uses machine learning to develop risk management tools to prevent fraud and money laundering in transactions.
Summing up, here are some benefits of ML for security:
- Minimising the risk of data breaches and cyberattacks by effective fraud monitoring.
- Real-time, effortless security monitoring that doesn’t require a lot of human input.
- Detecting and reacting to fraud transactions that usually can’t be identified by manually defined rules.
Machine learning for Anti-Money Laundering
Machine Learning (ML) plays a crucial role in Anti-Money Laundering (AML) efforts, enhancing the detection and prevention of illicit financial activities. ML algorithms are employed for transaction monitoring, analysing vast amounts of financial data to identify patterns and anomalies that may indicate potential money laundering activities. These algorithms can automatically detect and flag unusual transaction behaviour for further investigation.
ML models excel at anomaly detection by learning normal transaction patterns and identifying deviations from the norm. Unusual patterns, such as large or rapid transactions, transactions to high-risk jurisdictions, or changes in transaction frequency, can trigger alerts for closer scrutiny.
Furthermore, ML algorithms recognise complex transaction data patterns that may indicate money laundering schemes. This includes identifying layering techniques, where multiple transactions are conducted to obscure the origin of funds, or smurfing, which involves breaking down large transactions into smaller, less suspicious amounts.
ML also creates dynamic customer risk profiles by analysing customer data, transaction history, and other relevant information. High-risk customers can be identified more accurately, enabling banks to apply enhanced due diligence measures and allocate resources more efficiently.
Here are a few machine-learning use cases for AML: Google Cloud recently launched Anti Money Laundering AI. It provides a consolidated machine learning (ML) generated customer risk score as an alternative to rules-based transaction alerting. The risk score is based on the bank’s data, including transactional patterns, network behaviour, and Know Your Customer (KYC) data, to identify instances and groups of high-risk retail and commercial customers.
Machine learning for meeting compliance regulations
Financial businesses worldwide must comply with regulations to enter new markets, grow and compete. However, compliance sometimes feels like running an ultramarathon – regulations change even before you comply with previous ones.
The top 3 challenges financial institutions face due to ever-changing compliance standards are:
- Operational disruption
- Regulatory disputes and fines
- Reduced or slower innovation
Source: The Economist Compliance and Regulatory Disruption 2018 report
Quick fact: According to the Ponemon Institute, the average cost for organisations that experience non-compliance problems is $14.82 million, a 45% increase from 2011. Which is almost three times higher than the cost of compliance. And this number just kept going.
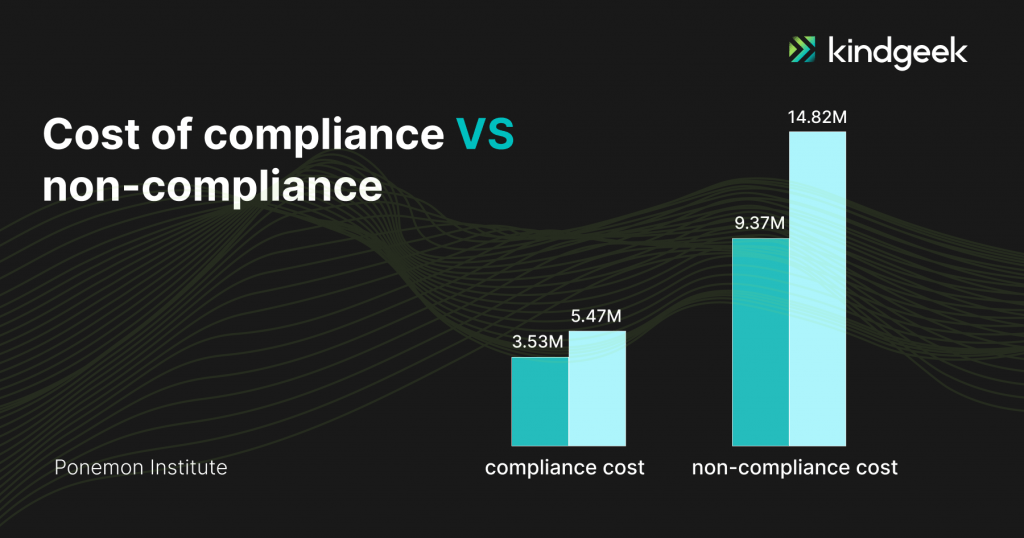
ML has become a salvation for many financial institutions trying to comply with unstable regulations. Machine learning algorithms can process large amounts of regulatory documentation and find correlations between the guidelines. ML-powered systems can automatically detect the changes in laws as they appear and immediately react to those.
Here are several startups worth mentioning:
Zest.ai helps lenders make better decisions and better loans — increasing revenue, reducing risk, and automating compliance. Zest.ai has raised $232 million in funding over seven rounds.
ComplyAdvantage is a UK-based startup that provides AI-driven financial crime risk data and detection technology to comply with security regulations.
Sym is a newly established startup based in San Francisco that solves the intent-to-execution gap between policies and workflows.
To sum up, here are some benefits of ML for meeting the regulatory requirements:
- Automated compliance regulations change monitoring.
- Minimizing time consumption for regulatory work by automating manual processes.
Machine learning for customer retention
Imagine a $100B bank dealing with hundreds of support requests every hour. Was my transaction processed? How do I increase my credit limit? How do I change the password?
The fact is that those requests are usually quite similar, and only some cases are exceptional and need real-time input from the support officer. Financial institutions can use machine learning to automate and fasten the support processes. With machine learning’s ability to delve into petabytes of data to find out exactly what matters to a particular client, financial institutions can benefit from providing personalised assistance and offers. What’s more – ML-powered solutions learn from previous experience and improve over time as they process more complex customer data.
One of the remarkable examples of machine learning in banking is chatbots. ML-powered chatbots provide real-time, client-oriented, and human-like assistance that enhances user experience and saves the company’s human and organisational resources. Moreover, those chatbots learn from each request; this way, conversations become more and more personalised and helpful over time. As a result, both small and large Finance businesses can benefit from incorporating chatbots – with less need to run customer support departments.
While chatbots can primarily benefit a company of any industry, Finance and Banking are the ones that can make the most out of their ML-powered “employees.”
Here are a few notable chatbot machine learning use cases in banking:
Wells Fargo was the first US bank to create an ML-driven customer assistant for Facebook Messenger. It was one of the first to develop machine learning applications in banking.
Bank of America was one of the first banks to introduce a virtual ML-powered assistant within a mobile app. Due to the pandemic, Erica added 1 million users a month from March through May 2020, bringing its user count to 14 million.
Kindgeek has also recently launched a new service – an AI virtual assistant. We offer technical advisory, GPT-powered AI assistant integration, and prompt engineering services to help you deliver superb customer experiences, enhance operational efficiency, and cut costs by automating your first-line customer support.
Why the time is right to deploy chatbots:
- The need for speed.
- The need for personalization.
- The need for data.
Machine learning in stock market forecasting
Just like the weather, stock market dynamics can be predicted. With lots of historical data, there is an excellent opportunity for developing machine learning-driven solutions to forecast stock markets. Large historical datasets combined with real-time data sources can be a powerful tool for predicting the stock market dynamics using ML. Machine learning can be used for any financial modelling, from determining future risks to predicting stock prices. The only thing left is building a system that would enable algorithmic trading. Here’s how it works:
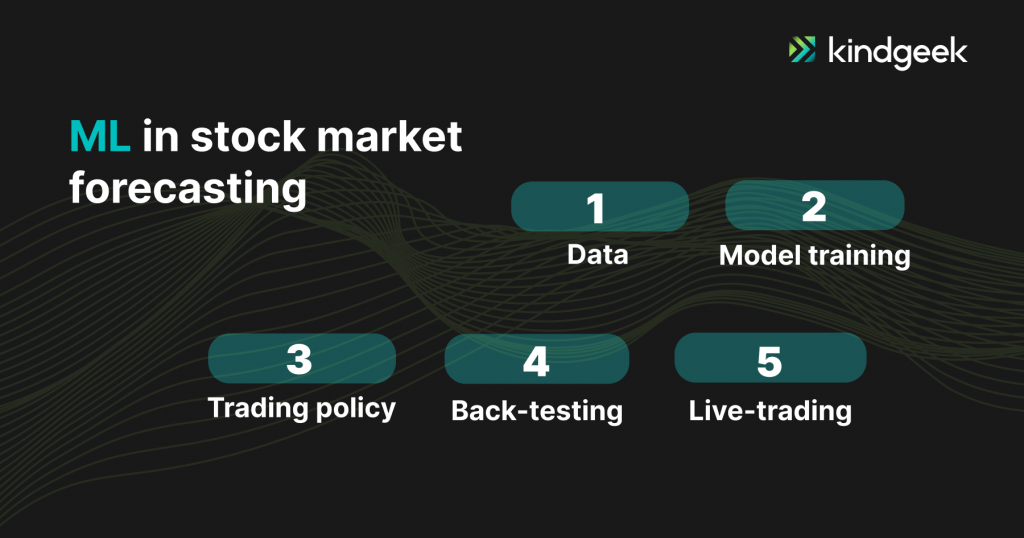
Many machine learning models are developed to forecast stock market prices. Many are designed to forecast cryptocurrencies, like SARIMA and FB Prophet. While some might find it extremely difficult to predict the future of Bitcoin, we can understand where it might go with a high degree of confidence in machine learning.
See some of the machine learning use cases for stock prediction:
Walnut Algorithms is a France-based startup that utilises AI and ML finance solutions for investment management.
QARA utilizes the latest deep learning technology to analyze and forecast the financial markets. The company also developed a mobile application.
Summing up, here are the key benefits of machine learning for stock forecasting:
- Machine learning can efficiently process large amounts of stock data from different periods and monitor real-time situations.
- Machine learning algorithms can notice even insignificant correlations to predict price changes.
- The reliability of the forecast is much higher than that of a prediction made by humans.
Machine learning for investment strategies
Machine Learning (ML) is a vital component of modern investment strategies, revolutionising the way financial markets are navigated. ML algorithms analyse extensive historical and real-time financial data to uncover patterns and trends that may elude traditional analysis. This data-driven approach enhances predictive analytics, giving investors more informed insights into future market movements and stock prices.
In the realm of algorithmic trading, ML is extensively employed. Automated systems execute trades based on predefined rules, learning from historical data to optimise trading strategies and capitalise on short-term opportunities. This capability allows for rapid responses to market changes, contributing to more efficient and adaptive trading strategies.
Another key application of ML is in portfolio optimisation. ML algorithms dynamically adjust portfolio weights and asset allocations by considering factors such as risk tolerance, return expectations, and market conditions. This optimisation aims to maximise returns while minimising risk, providing a more sophisticated and adaptive approach to portfolio management.
Market microstructure analysis, involving the study of order book dynamics, liquidity patterns, and transaction costs, all benefit from ML applications. This detailed analysis is crucial for designing high-frequency trading strategies and understanding the intricate dynamics of market behaviour.
ML is also employed in dynamic asset allocation, continuously analysing market conditions and economic indicators. This adaptability allows investment strategies to respond to changing environments, optimising the allocation of assets for maximum returns.
Here are a few machine-learning banking use cases for investment strategies:
BlackRock is one of the world’s largest investment management companies. Their Aladdin Wealth platform utilises ML to model individual investor behaviours and design optimal investment portfolios tailored to their unique preferences and risk tolerances.
Trade Ideas provides traders with an AI-powered robo-advisor consisting of several dozen investment algorithms subjected to over a million trading scenarios overnight to arrive at a subset with the highest probability for alpha in the next market session.
Machine learning for risk assessment
According to the McKinsey Global Institute, machine learning for risk assessment solutions could generate more than $250 billion in the banking industry.
For banks, machine learning can significantly fasten and lower risks for the loan approval process. Credit scoring is one of the most useful deep learning use cases in banking.
Credit scoring applications analyse data from rent payments, social profiles, telecommunication companies, bank history, and tax payments to make a complete picture of an individual requesting a loan in a few minutes. The algorithms compare the data of other customers and generate a credit score to indicate the risk.
Here are a few machine learning banking use cases for credit scoring:
Kreditech is a German startup that helps the company determine the creditworthiness of potential borrowers who do not have an extensive banking history using predictive analytics and likely natural language processing.
Aire provides credit assessment services that give people a new credit score to help them qualify for essential financial products.
Consider KindGeek, Your Trusted Machine Learning Development Partner
In 2016, we started as a company that delivers custom fintech software development services and has grown into a trusted finance software development provider. Our expertise helps us conduct an effective product discovery phase, develop innovative fintech solutions, and bring your ideas to life.
Read about our Fintech projects here.
Developing a fintech product from scratch is a time- and resource-consuming process. Kindgeek’s white label fintech platform may be a better solution. With a wide range of pre-built features, combined with thoughtful customisation and development, you receive a solution with the functionality to jumpstart your online finance business or embrace digital transformation without heavy upfront investment. We also provide a white label banking platform, which allows you to build on top of it and create a unique customer experience.
Final Thoughts
It’s already clear that AI is set to be revolutionary for Fintech and Banking. More so, those technologies are on their way to becoming irreplaceable for any financial institution to grow and compete locally and globally. Security and fraud detection, anti-money laundering, compliance, stock forecasting, investment strategies, risk assessment, client retention – all of that and more can be successfully done with ML.
As financial institutions increasingly embrace this transformative technology, the industry is poised for enhanced efficiency, data-driven decision-making, and a more robust compliance and risk management approach.
What are the benefits of machine learning for banks and customers?
With ML, banks can improve their security, fraud detection, risk assessment, and the quality of client service.
What are some use cases of machine learning in banking and finance?
Security and fraud detection, anti-money laundering, compliance, stock forecasting, investment strategies, risk assessment, client retention – all of that and more can be successfully done with ML.
How can machine learning in banking improve security?
With ML algorithms, the system can quickly recognise suspicious activity and send alerts to the security operations centre, improving the security of the financial organisation.